“The application process for the AITC program was characterized by its thoughtful approach, allowing applicants to comprehensively articulate the gaps in science/technology and innovative aspects of their research proposals. This thoughtful application process proved to be immensely beneficial not only for securing AITC funding but also for enhancing the quality and clarity of future grant applications, particularly those aimed at larger funding opportunities. Once the grant was awarded, we gained access to a wealth of invaluable resources. The AITC boasts a team of experts with diverse backgrounds and expertise, fostering a transdisciplinary environment that can address a wide range of project-related needs. These experts are well-equipped to provide support across various dimensions of a project, be it clinical, technical, or business/start-up-oriented. We gained significant knowledge around the details to translating a research idea into product.”
– Chintan Pandya,
Grant Year 1 Awardee
Alzheimer’s Disease and Related Dementia
The goal of the Alzheimer’s Disease and Related Dementia Pilot is to use a multidisciplinary, collaborative approach to identify, develop, refine, and disseminate promising technologies that have a high potential to improve the health and well-being of older Americans living with ADRD and/or their caregivers, with an emphasis on technologies that can mitigate current disparities in the access and delivery of dementia care. Pilot leadership’s duties include:
Year 1
An AI algorithm to screen for and detect probable dementia cases in the emergency department.
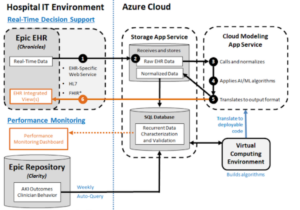
This project will produce a software product intended to aid in the detection of early mild cognitive impairment. Accomplishing this will assist clinicians on the front lines of early stages of dementia investigation, management, and treatment.
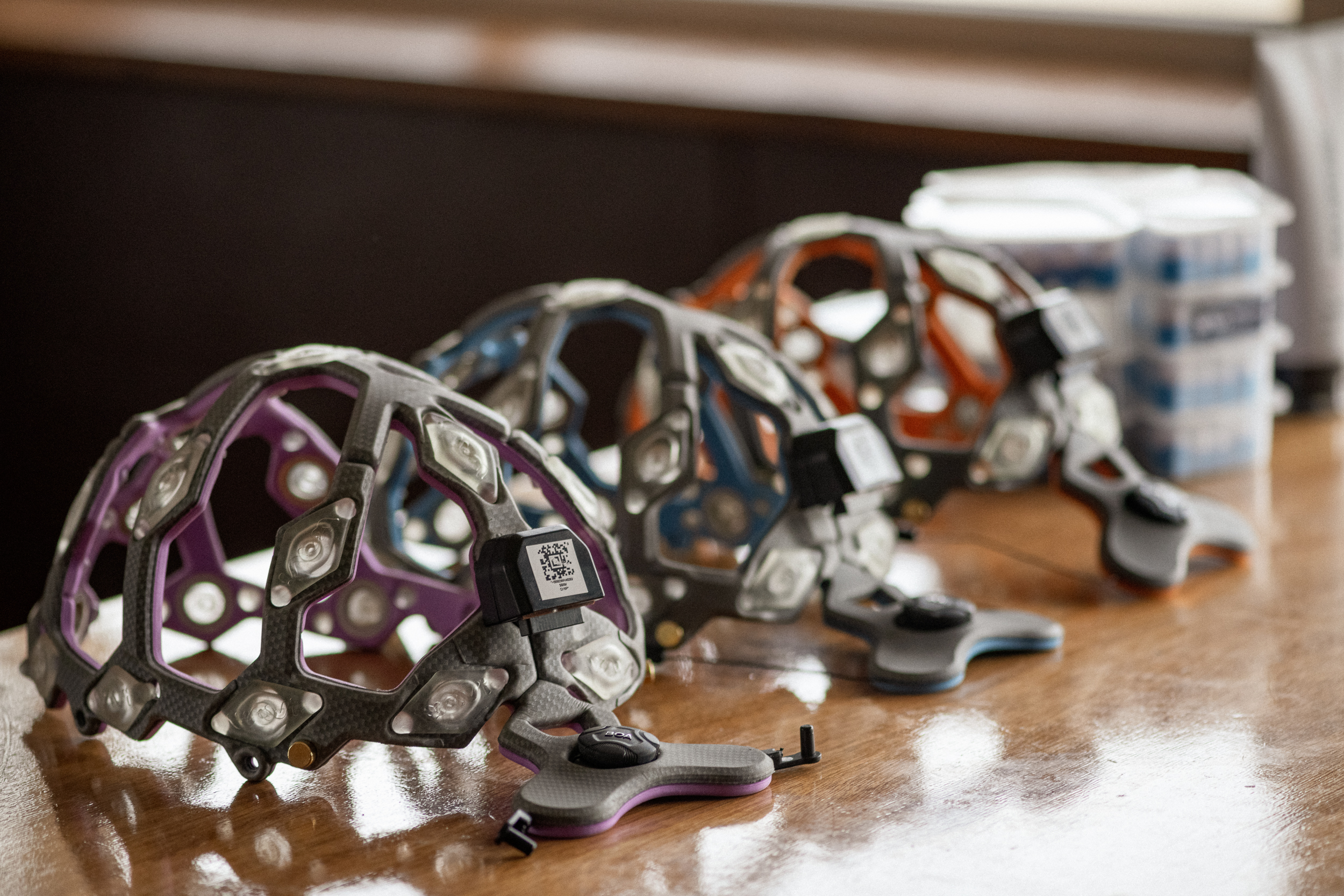
WellSaid.ai’s solution to address the need for early detection of cognitive impairment leverages simple-to-use, inexpensive, in-home consumer technologies like the Amazon Alexa platform, but equipped with scientifically validated, clinical-grade cognitive assessments modeled from gold-standard assessment batteries. This solution allows for the screening, identification, and clinical categorization of cognitive impairment and dementia well before an individual typically seeks medical attention and allows for the large-scale expansion of dementia screening and detection at an earlier stage.
WellSaid.ai’s pilot goal is to develop, test, and validate machine learning model(s) that accurately predict the cognitive status of older adults in their own home. The pilot has three main objectives: 1) Retrospectively analyze our existing datasets to guide model development; 2) Prospectively collect comprehensive cognitive assessment data, including raw audio data from 160 patient-caregiver dyads from our in-home assessment technology; and 3) Develop predictive models using natural language processing and supervised learning algorithms that can identify and predict cognitive impairment.
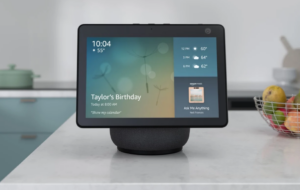
People Power, now doing business as Care Daily, will develop and research an AI caregiver service called “Arti” that receives data from Apple watches and sends useful alerts, recommendations, and encouraging messages to caregivers watching over people with health problems such as Alzheimer’s disease. “Arti” should be able to alert on falls, predict falls before they occur, detect wandering, and alert caregivers of the GPS location of a missing person with Alzheimer’s, monitor their sleep quality trends and offer recommendations for improving sleep quality, monitor their fitness trends, recommend ways to improve caregiver health, and provide other senior care microservices.
The Sovrinti in-home system combines device utilization and real-time location information to monitor seniors and their caregivers in the home and assisted living environments. The system’s rich sensor data allows for the continuous identification of activities of daily living and and instrumental activities of daily living.
For this project, Sovrinti will utilize AI techniques with de-identified data from an ongoing National Institute on Aging Phase II study to preemptively identify adverse effects in seniors with cognitive challenges. This project will utilize the hundreds of months of available sensor data and associated monthly health status reports to develop and validate the use of the Sovrinti system to predict rising risks of specific adverse events associated with senior care. These adverse events include urinary tract infections, COVID-19 events, falls, hospitalizations, and more.
This approach combines an anomaly detection component with a trained embedding for each home or care recipient to create a risk prediction model; the risk prediction model will be used to establish alerts for caregiver intervention. In addition to model development, validation will establish confidence parameters for alerts and quantify confidence level as a function of days prior to an adverse event, with a goal of high confidence alerts occurring 2–5 days in advance of an adverse event.
This project will apply a deep learning framework to develop a prediction model that can be commercialized as a service offering personalized cognitive impairment risk prediction. Toward precision medicine and care, a deep-learning-based brain MRI analysis will be applied to provide an objective measure with which to quantify the risk for the development of cognitive impairment and identify modifiable factors that could accelerate or slow the pathological processes for each individual. This study will provide clues for disease prevention and therapeutic interventions.
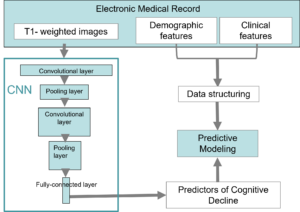
Sequoia is developing a wearable technology that makes acoustic stimulation accessible to older adults in the comfort of their own homes. By adapting this technology to the unique needs of older adults and packaging the complex sensors, circuitry, and algorithms required for acoustic stimulation into a comfortable and easy-to-use headband, we are translating this laboratory technique into an accessible therapy. This developmental work will entail an exploratory usability study to thoroughly understand essential design features. The resulting information will be used to focus our prototyping efforts as we continue our algorithm development. To validate the results of this work, we propose a small clinical feasibility evaluation of our prototype device.
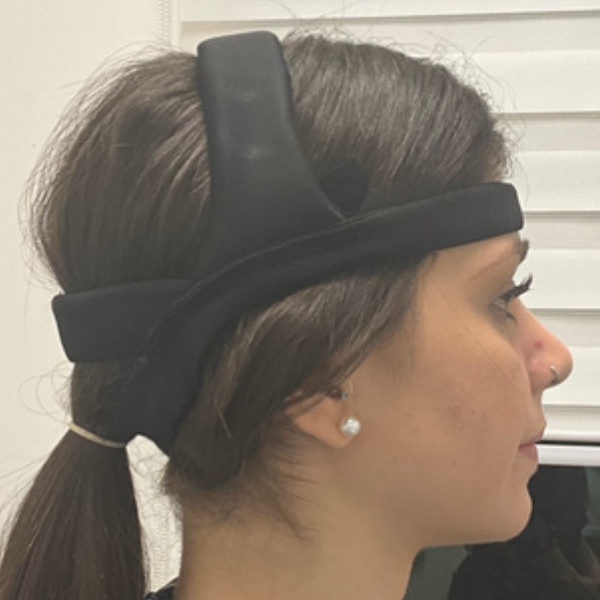
Lucidity is a mobile technology platform for home use that reports cognitive changes in an individual with Alzheimer’s disease to their caregiver. The system will also provide a framework for quantifying cognitive fluctuations and lucid intervals in individuals with Alzheimer’s.
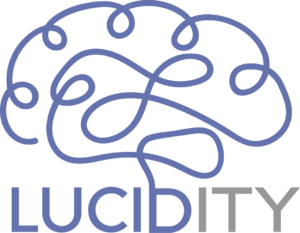
An AI-based facial expression analysis software will be integrated with an autonomous navigating robot to visit nursing home residents with advanced AD/ADRD and detect their agitation. This can open opportunities to deliver non-pharmacologic interventions and to improve quality of care.
This project will apply AI analytics and electronic health records to develop/disseminate prediction models to identify persons with ADRD who would benefit from palliative care. The tools developed will support resource planning, quality and equity assessment within health delivery systems, health plans, and communities.
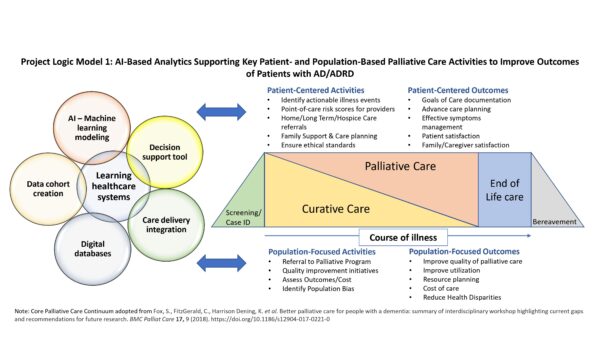
This is a virtual reality world for people with dementia that delivers therapies and activities of daily living engagement. This project will build with a AI-based dialogue management system to make it more interactive and to allow caregivers more downtime.
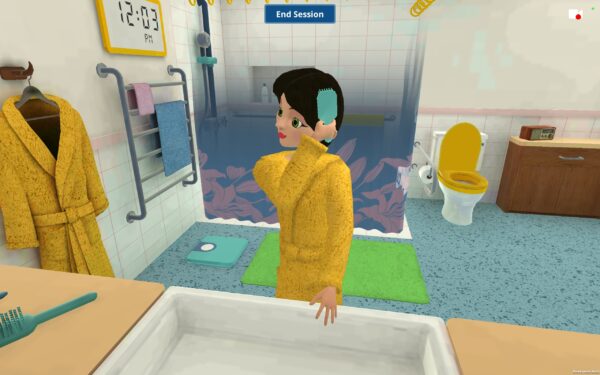
Year 2
To advance the use of digital technologies in diagnosing early stages of Alzheimer’s disease and mild cognitive impairment, we propose a research project using voice recordings, neuropsychological tests, neuroimaging, and neuropathology data. By developing interpretable deep learning models, we aim to identify cognitive abnormalities in speech that align with brain atrophy patterns and neuropathology grades. The project’s success will provide a validation framework for researchers and expand the application of voice technologies, making them accessible to diverse populations.
EZ-Aware is an easily accessible digital health platform that uses active and passive monitoring of cognitive and behavioral functions in older adults’ everyday environments. Current cognitive screening tools to detect mild cognitive impairment (MCI) are time-consuming, are not used routinely, do not assess functional abilities in daily life, and are not sensitive to the earliest signs of neurodegenerative disease. To overcome these current barriers and limitations of cognitive screening in older adults, the goal of this pilot project is to design a functional version of EZ-Aware that will be piloted for feasibility among cognitively healthy individuals and those with MCI. All study data will be used to form a digital twin model, or a digital representation of each individual participant in the study.
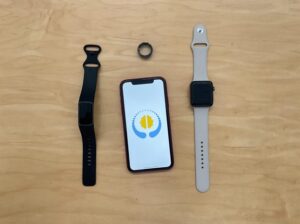
Emerging data suggest COVID-19 increases the risk for or potentiates the onset of Alzheimer’s disease in older adults. Those living in underserved urban and rural communities face distinct challenges in accessing dementia care. There is an urgent need to identify novel methods to evaluate the impact of COVID-19 on the cognitive health and dementia risk of older, at-risk adults, and to do so in a manner that facilitates access for those in low-resource settings.
The DANATM Cognitive Assessment Tool is an FDA-cleared mobile application developed and validated to detect subtle cognitive dysfunction. The study team has successfully partnered with DANA developers to track cognitive trajectories following COVID-19. In this prospective cohort study, older adults will complete the DANA battery on multiple occasions following the contraction of COVID-19. By applying machine learning techniques to the in-home, app-based measurement of cognition, this project will develop a more accurate, reliable, and accessible method of detecting and predicting cognitive decline and Alzheimer’s risk following a COVID-19 diagnosis.
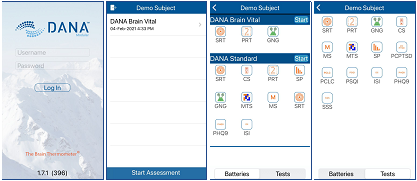
The EyeControl platform is designed to support patients in the ICU to improve communication between patients, families, and medical teams and to support the management of delirium. This project will evaluate the efficacy of the EyeControl platform specifically in older adults admitted to the Johns Hopkins ICU and pilot new device features for delirium management.
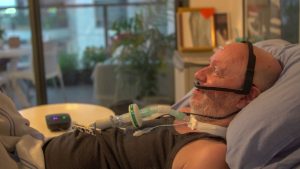
This project will develop methods for accurate and non-intrusive assessment of neuropsychiatric symptoms (NPS) at home over time, document the sequence of occurrences of NPS, predict adverse events, and provide interpretable data for accurate diagnosis. We will develop a clinician dashboard summarizing patients’ trajectories of NPS-related behaviors and correlate these measures with the onset of dementia. The goal of this project is to build the infrastructure for a large-scale clinical trial.
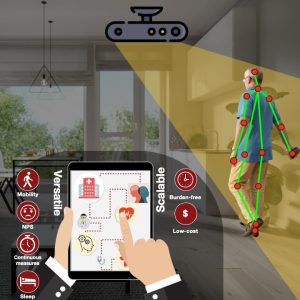
Healthy Aging
The goal of the Healthy Aging Pilot is to utilize a multidisciplinary collaborative approach to identify, develop, refine, and disseminate promising technologies that have high potential to improve the health and well-being of older Americans and/or their caregivers, with an emphasis on technologies that can mitigate current disparities in the access and delivery of health care in rural and urban areas across the US.
Year 1
Virtual Apprentice, a women-owned VR content development company, has developed a prototype platform, ReTreatVR™, designed to provide senior-friendly immersive experiences to support positive aging. Prototype experiences are designed to create happy memories, re-engage with the world, and enhance social interactions that can be shared and discussed with others. This project will support positive aging and reduce social isolation.
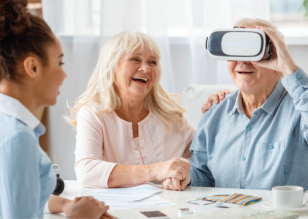
A multidisciplinary team of ophthalmologists, engineers, and entrepreneurs at Johns Hopkins University have developed a simple and inexpensive anterior segment imaging and telemedicine system to allow for remote eye screening facilitated by non-ophthalmologists. The system will be applied to address disparities in cataract screening, referral, and disease management for older adults in the United States. Partnerships will be formed with ophthalmology practices, senior living facilities, and long-term care facilities to further develop this platform and incorporate artificial intelligence in order to provide simple, accessible, and near real-time diagnosis and referral of vulnerable older adults with age-related cataracts.
PI: Kunal Parikh, Research Associate in the Department of Biomedical Engineering at the Whiting School of Engineering and the Johns Hopkins School of Medicine
This project features a handlebar device that can be used to “train” seniors to improve their balance. The project will perform user testing of the Balance T device, a mechanical device designed to improve static and dynamic balance. The Balance T device will be provided to volunteer participants age 60 and above who are interested in improving their balance. The project will administer surveys to be completed post-use to assess usability of device, adherence to device use, and self-reported changes in balance.
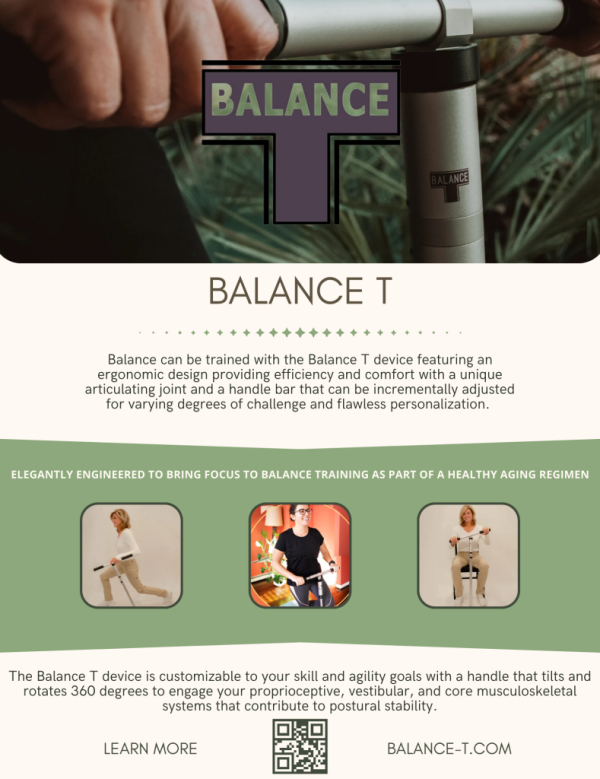
Year 2
Physical function, or the ability to perform daily activities, is important to screen among geriatric patients, since it can determine one’s independence and is linked to frailty, disability, and death. However, time and space constraints, lack of trained staff, and limited reimbursement mechanisms make it difficult for providers to screen for physical function. Additionally, patients are unable to accurately and consistently measure their own physical function; therefore, their treatment plans will be affected.
However, wearable devices can collect mobility, balance, and gait data and can incorporate software to analyze physical function accurately without the need for supervision. We developed a Geriatric Function Assessment (GFA) system that effectively evaluates physical function among older adults. The GFA system will be incorporated into a wearable device that will perform motion and visual monitoring as patients navigate among common clinical areas during clinic visits. We aim to optimize the GFA system’s design and calibration, generate deep neural network models, and perform a feasibility design among 21 older adults with multiple chronic conditions in a clinic-based setting to maximize the user experience. We envision that the development of this system will provide a solution to both clinicians and geriatric patients by producing accurate physical function data in an efficient manner.
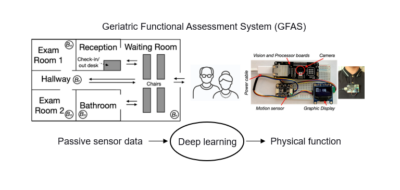
Falls are associated with immobility, mortality, and decreased independence, leading to social isolation and reduced quality of life. One of four individuals over 65 years old sustains a fall each year. While predictors of falls have been identified—including fall history, age, and cognition deficits—these data fail to provide insight as to how to treat or track fall risk. As the aging population increases, the economic burden for hospitalization and supervision for those at risk for falls rises. A critical step toward improving our ability to treat fall risk is to identify technology to efficiently collect clinically targetable and fall-relevant outcomes both in the clinic and the home.
To achieve this goal, a novel, portable, and inexpensive shoe insert has been developed to measure standing and dynamic balance outcomes. The smart insole provides balance and gait parameters in real time, providing the opportunity to collect highly quantitative fall predictors in the home or the clinic and thereby facilitating the design of specific interventions to prevent future falls. The purpose of this project is to validate this insole technology and relate it to reported falls. Walking assessments will be conducted on 50 individuals with and without these insoles and on a subsample of individuals wearing insoles in the community. Focus groups will provide input from health professionals and insole users. Collectively, this project will allow us to 1) validate insole outcomes to in-lab gold standards, 2) validate the clinical utility of outcomes by relating them to falls, and 3) validate the clinical usability of these outcomes by stakeholders.
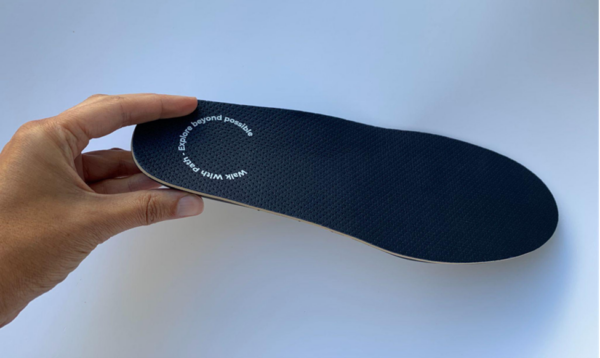
This pilot will capture patient balance data using a force plate at the point of care in a geriatric outpatient clinic to provide insight into what medical conditions and social determinants of health influence balance and the underlying risk of injuries for older adults. A large registry of balance data is key to using machine learning to aid in clinical assessment though a real-time dashboard. This will allow for the identification of appropriate treatment options, as well as the implementation of fall prevention strategies to create solutions and shift the focus from treatment to prevention.
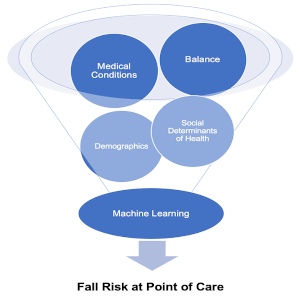
This project will leverage our lightweight and affordable exosuits in concert with learning-based personalized control to promote physical activities in everyday settings and explore the potential to ultimately decrease the risk of cognitive decline which leads to dementia in older adults.